Data Engineering Vs Data Science- What’s The Difference And Why You MUST Know It
- May 21, 2019
- Posted by: admin
- Category: Data Science
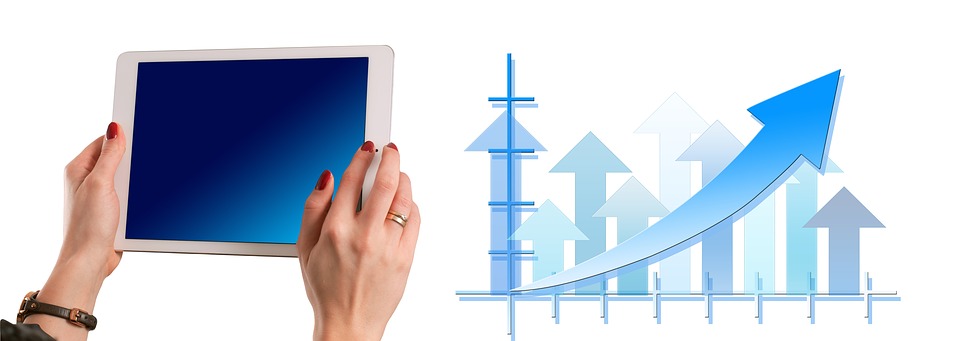
As technological innovations are making their way into the world, new job profiles are being created every day. With new roles and jobs surrounding these highly advanced streams, it is natural that there will be an ensuing confusion.
Among the latest developments in machine learning that have surfaced today, data engineering and data science are grabbing major attention. Although both are closely related to machine learning, the job responsibilities of each are pretty much diverse, and the professional skill sets required also vary.
With an intent to clear out the general puzzlement that prevails around Data engineering vs. Data Science, this will be a good read.
Differences between a Data scientist and a Data Engineer
Every sector in the industry is recognized by the skills, knowledge and hard work of its professionals, and in this respect, machine learning is also not an exception. With so many skills, there is, of course, some overlap in the jobs too.
So, without feeding to your confusion, here is the chief difference between a data scientist and data engineer:
Data engineers work to create the perfect infrastructure and environment required to generate data. After the data is successfully generated, data scientists implement statistical analysis techniques and advanced mathematical formulae on that generated data.
Although data scientists are constantly supervising the efficiency of the data infrastructure that is designed and maintained by data engineers, they play no part in setting up this infrastructure. You can consider them more like the team that is entrusted with the chores of conducting deep analysis and research for the sole motive of identifying patterns and trends in user data.
Interestingly, this is the perfect scenario in which you would find how data engineers are actually supporting data analysts and scientists with their skills. They are the ones who provide the solutions which when applied in the right way, helps to smoothen out complex business problems and open up market insights.
- The role of data engineers includes creating a robust and high performance which triggers certain actions. For instance, data engineers draw actionable insights from raw data and apply complex analytical tools with the aim of collecting and analyzing data. Then they organize it all into batches so that data scientists can carry on with the process tasks.
So, in a nutshell, without data engineers, data scientists cannot fulfill their job responsibilities. Without that perfect data infrastructure, neither would they be able to access data nor apply statistical methods for the benefit of enterprises.
- A data scientist should be adept in analytical tools like Hadoop, R, SPSS, and other advanced statistical procedures whereas a data engineer is expected to be knowledgeable in designing the interface that is needed to support these tools.
Now, here is a simple analogy of the two job roles, to help you get a crystal clear picture.
Data engineers are said to be the plumbers of data pipeline and data scientists are the painters who tell the story of this data meaningfully.
How do Data Science and Data Engineering complement each other?
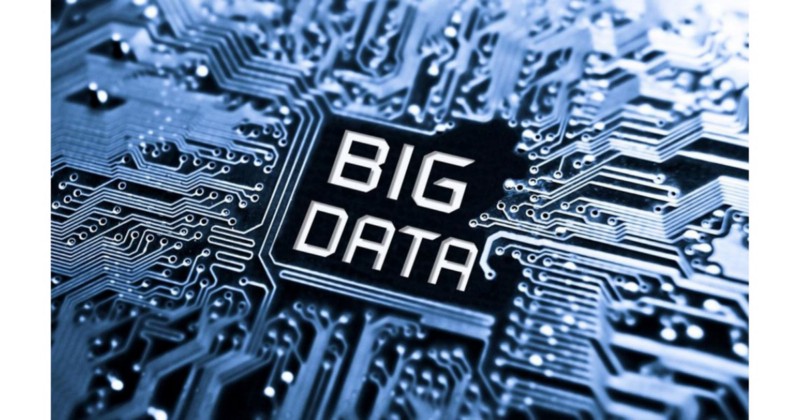
You might already be aware of the fact that Big Data is no longer a luxury for companies. It has, in fact, turned into an absolute necessity nowadays. In such a scenario, both data scientists and data engineers are equally important. So it is needless to clarify further that a Big Data professional cannot function both as a data engineer and a data scientist at the same time.
But while the departments of data engineering and data science vary on certain parameters, both the sectors actually function together, parallel, thereby, forming a close-knit team.
The skillset needed to be a data scientist should well complement the skills needed to be a data engineer, to ensure smooth functioning of Big Data capabilities in an organization. And for this to happen, it is also extremely vital for the departments to communicate with each other to remain resourced and updated. Data science and data engineer teams need to primarily recognize and understand how the handshake occurs between them. This is the first step to guarantee zero human error in handling the data pipeline.
What are the skills desired in Data Science?
Employees generally belong to applied mathematics or statistics background with computer science as a mandatory subject. Data scientists also need to ace their communication abilities since they need to interact with business analysts to a considerable extent.
What are the skills desired in Data Engineering?
Generally, a system engineer belongs from a computer programming background, and with these skills they design the data pipelines.
Conclusion
To sum it all up, in spite of various differences in Data Engineering vs Data Science, ultimately the overlap that occurs shows that both streams go hand-in-hand. Without one the other becomes totally incapable.