- March 2, 2022
- Posted by: Aanchal Iyer
- Category: Artificial Intelligence
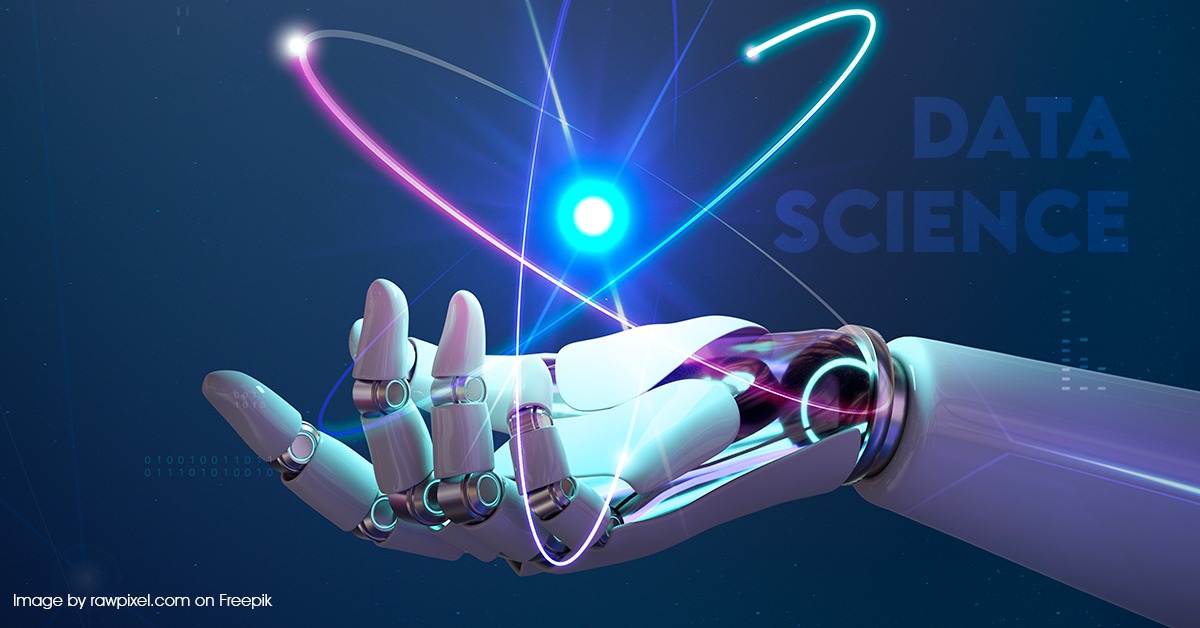
Data Labelling for AI: Important Considerations to Accelerate Quality
Introduction
Data Labelling for AI
Latest reports state that the global Artificial Intelligence (AI) industry will reach close to $190.61 billion by 2025. The adoption and use of Machine Learning (ML) and AI have resulted in the heavy requirement of data annotation, with an anticipated growth of 32.54% CAGR by 2027. Developing an ML/AI model needs vast amounts of training data and the biggest challenge in accessing high-quality training datasets. Data quality is the main reason why AI projects fail, succeed, or exceed budgets of ML and AI organizations.
ML and AI algorithms learn from data labelling, which makes data labelling one of the most vital parts of algorithm development. Data labelling, also termed as tagging, data annotation, or classification, is the process of creating datasets for algorithms that identify repetitive patterns in labelled data.
The success of ML and AI applications completely depends on the data quality and data, which is why close to 80% of the time spent in an AI project is on data labelling. One needs to identify their project requirements, understand the volume of data required, organize and clean the data, set up a quality check process, and establish a workflow. High-quality data is a requirement for the success of ML and AI models and it is essential to understand how to collate and prepare the data for adequate data labelling. Poor quality data leads to flawed AI models.
What Affects Data Quality
The data quality also drops due to the challenges faced with processes, workforce and technology. Lack of contextual understanding and domain knowledge leads to accuracy issues in labelling. Moreover, since Machine Learning is an iterative process requiring multiple tests and model validation, the workforce also must be agile.
Adjusting to continuously changing workflow based on validation and tests is crucial for high-quality data labelling. And then, selecting the right data labelling tool is essential to enhance the quality. Finally, the dataset must have good variety and balance for the algorithms to foresee similar patterns and points.
Considerations to Accelerate Quality Data Labelling for AI
Following are the major considerations to improve quality by optimizing data labelling accuracy and efficiency for AI.
-
Balance data points for algorithms to predict better
: There are multiple annotations depending on the type of data, such as audio, text, image, video, and so on. Based on the business goal, recognize the data that need annotation. Keep data diversified to be able to refer ML models in multiple real-world scenarios. Be sure of your requirement as each case requires a specific approach.
-
Optimize and ensure data quantity needed to train multilevel marketing
: After identifying the data type, understand the data quantity. Based on project requirements, fix the amount of data required. Huge quantities of quality training data enable machines to understand better. Thus, annotated data makes the device smarter. For any ML project, vast volumes of data need to be labelled.
-
Data quality for the success of ML models
: Before beginning any AI project, do ensure that the data is clean. Data cleanliness plays a crucial role in labelling. Data from multiple unstructured and structured sources may have discrepancies. Experts in the field use automated data cleansing technology and tools that have solutions to prepare data for training.
-
Measure training data quality through QA process
: For a ML model to work successfully, the labels on data need to be unique, accurate, and informative. QA ensures that data meets all these requirements.
Conclusion
Data quality is the biggest challenge in data labelling with challenges such as maintaining a specialist workforce, manual processes, finances, and so on. Businesses are required to consider an automated approach for accurate and quick labelling. With significant leaps in processes and technology, everything is more streamlined.