- December 20, 2019
- Posted by: admin
- Category: Deep Learning
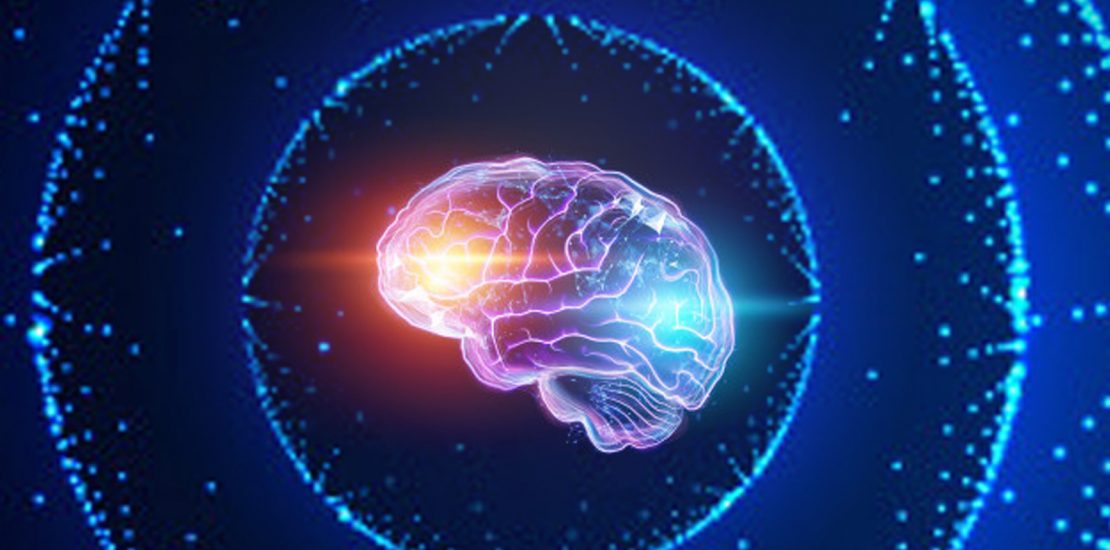
Fluorescence microscopy is priceless for cell biologists and researchers in identifying microscopic cell structures from images. However, this technique comes with certain limitations, which were observed by biomedical engineers.
For example, there are restrictions on how many fluorescent tags can be introduced in a cell structure. Apart from this limit, there are other side-effects observed, such as photo-toxicity i.e. the toxicity or damage from continual exposure to light. Phototoxicity can easily disturb the scientist’s work while they are trying to conducting live-cell imaging.
Upon coming across these limitations of fluorescence microscopy, a team of researchers started working on a way that would help them identify the parts of a living cell structure at a deeper and clearer level. They adopted brightfield microscopy, which was much more affordable than fluorescence microscopy. However, that also turned out to be less effective because of the grayscale images that were generated.
What did the team do to overcome the limitations?
They decided to develop a method so that they can identify a living cell’s components. To overcome the limitations of both techniques, the team employed technology to help them determine changes in the spatial organization of cells when they moved from one state to another.
That is when they adopted deep learning and AI to identify patterns from existing datasets.
For this purpose, researchers decided to turn their focus on developing advanced Deep Learning Algorithms that can leverage Convolutional Neural Networks which are highly effective in analyzing and classifying images. Their primary aim was to identify the similarities that lie in both fluorescence microscopy and brightfield microscopy images of the living cell structure. Images of various cell components like mitochondria, cell membrane, nuclear envelope, and others were used for analysis and generating clearer and more accurate images using deep learning.
What advantages did deep learning offer here?
These advanced AI algorithms, after comparing several pairs of images, eventually display the locations of structures that were fluorescently labeled earlier. But the major upgrade is that now, the same things were being represented in 3D brightfield image of live cells. The researchers also came to the conclusion that the newly found predicted labels were highly correlated with the actual fluorescent labels for several components of the living cell.
Researchers assert that with the help of Deep Learning applications, they were able to derive a beautiful time-lapse of all the cell parts moving around and interacting with each other in real-time.
Contrary to popular assumption, the Deep Learning concepts adopted here do not require thousands of images to learn. In fact, it works perfectly with just a handful of images that are easy to collect. So the overall process of imaging has become much faster than before.
Are there any limitations to the AI approach?
The only limitation of using deep learning to identify cell structures is that it is primarily expensive. But with more research, this is expected to become more affordable in the future.
Deep learning is expected to contribute on a massive scale for further research in fields like pathology, to quickly identify ailing cells from the changes in cell structure.