How can We Make Predictive Analytics More Efficient with AI?
- January 7, 2022
- Posted by: Aanchal Iyer
- Categories: Artificial Intelligence, Predictive Analytics
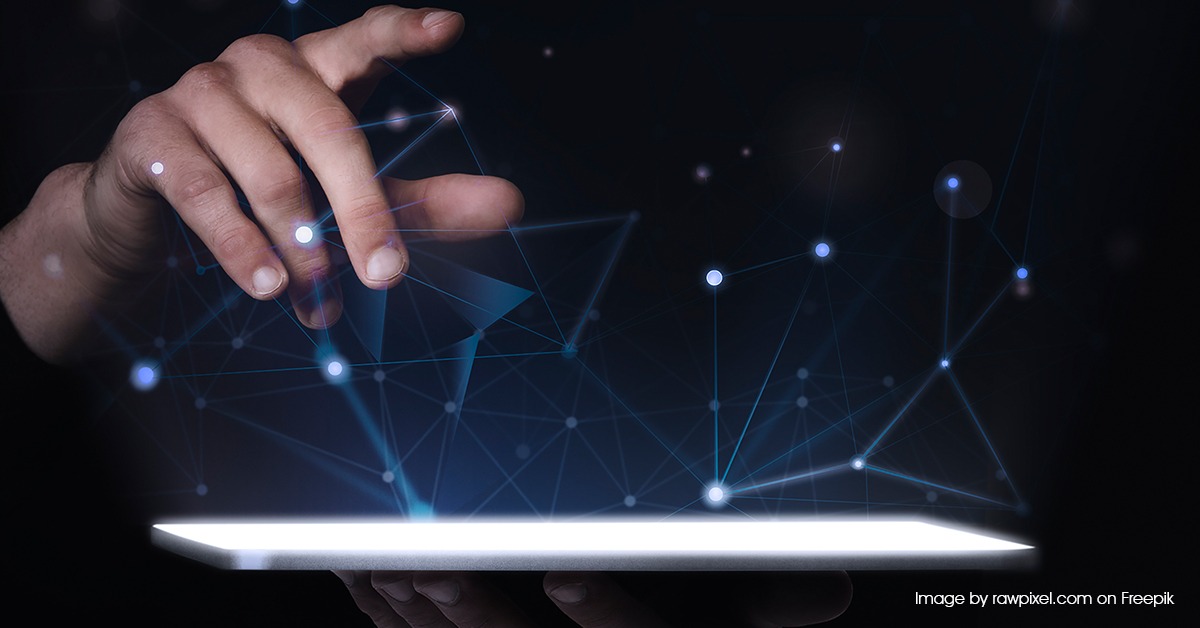
Predictive Analytics More Efficient with AI
Introduction
As the supply chains stabilize globally, most manufacturers are returning to usual operations with advanced and robust technological abilities. During the pandemic, nearly half of the supply chain leaders increased their expenditure on advanced systems and technologies. And this includes predictive analytics.
Predictive analytics uses statistical algorithms and external and internal data to predict future trends. This enables organizations to enhance inventory, delivery times, sales and eventually, decrease operational costs. When coupled with Artificial Intelligence (AI), the insights gathered from such advanced systems are vital to more accurate and timely forecasting.
When We Cannot Depend on Historical Data
Predictive analytics enhance processes using Machine Learning (ML) and historical data such as consumer behavior, weather patterns, and gas price variations. However, what happens when historical data can no longer predict the future?
Take the example of the Covid-19 pandemic. This is one disruptive event that devastated the global economy and twisted predictions. Supply chain leaders using predictive analytics could never have accounted for the global economic shock resulting from the pandemic.
For example, businesses that manufacture toilet papers or PPEs could not forecast the demand for those products during the pandemic. Meanwhile, restaurants, small businesses, and service providers had no easy way of altering their inventories and operations to match the demand.
Consumers’ behavior and purchasing trends during the pandemic were not prognostic. Businesses were struggling because of the anomalies in consumer behavior, and the decision to even take in data from 2020 in predictive models is arguable.
There will always be remote factors that twist data. But the more data sources one has, be it internal or external, the more accurately one can predict. However, this data needs to be paired with AI and predictive analytics. The challenge in such a scenario is knowing where to find the data.
How AI and Predictive Analytics Can Optimize Supply Chain Efficiencies
Pairing AI with predictive analytics models is essential to improve the forecast accuracy post-pandemic. In practice, this means having up to the second data for each resource. For example, a lack of raw materials can affect plastic supplies due to unexpected shipping delays or natural disasters. An AI system can flag likely events in advance, thus, resulting in a more informed decision-making.
To enhance predictive analytics, the following methodologies should be considered:
- Inventory management: one can optimize the inventory management beyond simple reordering by syncing real-time data with AI. When data such as every single barcode scan is fed into an analytics/AI engine, then this data can provide insights into the patterns of the inventory movement,
- Delivery optimization: Predictive analytics has enhanced trucking routes and ensured on-time deliveries. Real-time rerouting can be enabled by pairing route optimization software with AI. However, this depends on various factors. In addition to route optimization, IoT devices can collate real-time sensor data on trucks to enhance operational aspects of deliveries.
Unlocking Actionable Insights Through Data
The recent pandemic highlighted the power of AI paired with predictive analytics paired. Data collection is vital in the supply chain, but it is useless if it does not lead to action. We are collating more data than ever. However, AI is required to transform it into actionable and predictive insights. To get started, one needs to have a good plan and team buy-in to really start capturing the data points and the suitable technology on one’s journey towards fully implementing predictive analytics using AI.