How COVID-19 Impact On Data Analytics Models
- June 20, 2021
- Posted by: Aanchal Iyer
- Category: Big Data Analytics
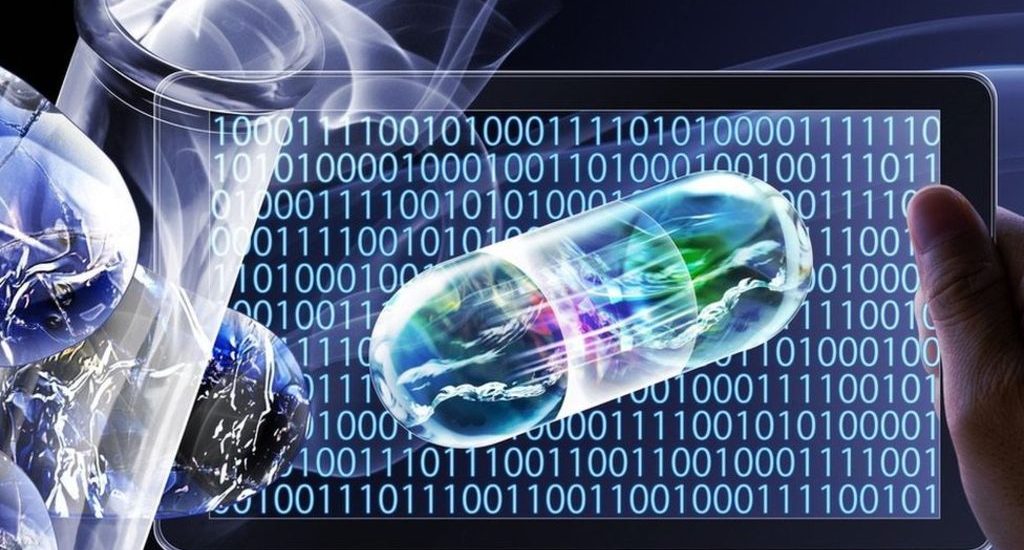
Impact of COVID-19 on Data Analytics Models
AI and advanced analytics are priceless to companies managing uncertainty in real-time, but almost all predictive models depend on historical patterns. How can companies account for an emergency and get more from their analytics strategy during such times?
How has COVID-19 Impacted Organizations?
The COVID-19 pandemic has augmented most companies’ use of AI and advanced analytics. These policies have assisted in engaging customers through digital channels, handle delicate and multifaceted supply chains, and assisted workers through disruption to their lives and work. At the same time, leaders have recognized a key weakness in their analytics strategy: the dependence on historical data for algorithmic models. From user behavior to supply and demand cycles, historical patterns and the assumption of continuousness are what provide predictive models their power. COVID-19’s impact on how we live, work and function has changed those patterns—and the models that organizations use for making business decisions. Still, these models have great value.
Firms are Reverting to Descriptive Analytics
Organizations have settled for predictive analytics in the last several years, as they use data to anticipate future requirements and trends. However, forecasting demand is tough even in normal times, and the unpredictability due to the pandemic has been challenging. Since the pandemic started, executives have side-lined predictive analytics programs and gone back to simple descriptive analytics — good data about the present and recent past that is swiftly accessible.
However, there will likely be other pandemics in the future, as well as disasters of other kinds. There have also been similar unsettling events in the past, such as the 2008 economic crisis. The need of the hour is strategies for analytics models that will help organizations with the new normal.
Analytics Models and Strategies
Following are some analytics models and strategies on track for the next normal:
- Increase the company’s data sources: Companies should concentrate on where they can gain new insights instead of depending on lagging information. These can come from both from new sources of data and utilizing prevailing data in new ways. For instance, movement data from cell phones can offer a clearer picture of economic activity by location.
- Improve decision-making processes: Organizations cannot let their models run in an autopilot mode; to gain the most value from them, leaders often need to expand models with human judgment. Analytics leaders must also reconsider how we learn from history and weigh situations in a different manner based on their context. Simpler models, which are more explainable and transparent, also help.
- Introduce scenario analyses: Businesses should now plan for a wide range of scenarios rather than a solo prediction or baseline outcome. For example, when it comes to a functional end of the pandemic, companies must plan for various factors, including vaccine progress and distribution, satisfactory levels of immunity, and the progression of various therapeutics. All these forces will impact how people function and work, and they are likely to unfold at various speeds in different geographies. Businesses that model a range of scenarios will be able to prepare better and plan.
Advanced analytics has enormous benefits for rapid decision making, but companies need to question their existing models, make adjustments, and remain agile.