Learn How to Avoid Fatal Data Science Mistakes
- June 15, 2019
- Posted by: admin
- Categories: Big Data Analytics, Data Science
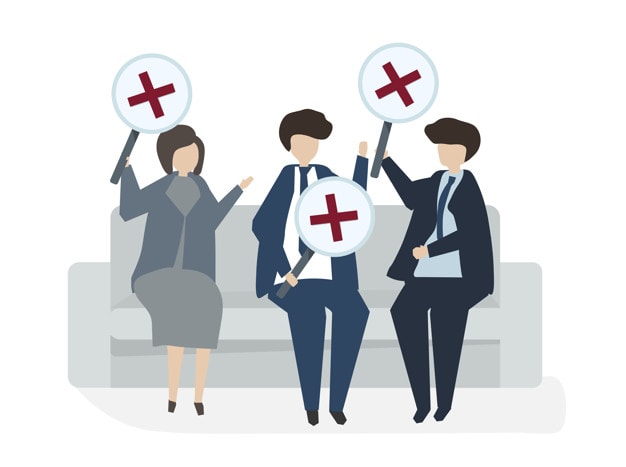
Data Science is one of the most advanced innovations in technology. Although mind-blowing advancements have already been recorded, this field is still in its nascent stage. In spite of its ongoing evolution, data scientists are of the opinion that the power of data science is such that it can make or break a business. So the margin for Data Science mistakes is really too narrow.
Does that mean that Data Scientists never make a single mistake?
Well, even if they do, the chances are rare; besides, such errors can be avoided at all costs. Let us find out some of the common mistakes in Data Science and how they can be prevented.
Common Mistakes in Data Science
1. Cherry Picking:
It is human nature to choose and pick whatever looks best when we have a range of options in front of us. But in the case of data science, user data cannot be treated in this way. No matter how good or bad user data looks, every single detail counts. If we start picking the good data from a set just like good-looking cherries, it will ultimately project a wrong picture of the overall performance of a business. Poorly represented data can lead to wrong business strategies which can cost businesses an average of $9 million annually, as per Gartner. (Source)
To avoid this fallacy, data scientists abide by honesty and impartiality. The key is to be objective and base the solutions on data as-is.
2. Correlation-causation Confusion:
It has been observed that many data scientists tend to assume that causation and correlation are the same things. While it is good to inspect the correlation between two variables using big data, it is not always correct to make it analogous to a cause-and-effect model. While correlation reveals the behavior of two different variables in the same span of time, it is not always mandatory that one is the cause of the other. When correlation and causation intermixes, it might lead to irreversible data science problems that impact businesses negatively.
So it becomes extremely important to clearly understand the differences between these concepts and then analyse data.
3. Analysing Without a Plan:
The entire domain of data science is highly structured and based on innumerable aspects that are clearly defined. Of course, there are certain hypotheses too, based on which the decisions are charted for fulfilling objectives. Data science projects that lack a line of action, invariably yield results that are ineffective. Overlooking possibilities and missing out probable scenarios while analyzing data can often lead to incomplete investigation and confusing outcomes.
In order to achieve the goals of a particular data science project, the primary step should be to chart out the overall path of analysis that will lead to the desired outcome. Data science experts leave no questions unsolved in their analysis so that risks can be effectively mitigated and businesses can yield higher profits from data science.
Conclusion
These are only a few of the common big data challenges in 2019 that experts have recorded. But with a well-defined plan and adequate alertness, all of them can be well prevented.