- February 3, 2023
- Posted by: Aanchal Iyer
- Category: Artificial Intelligence
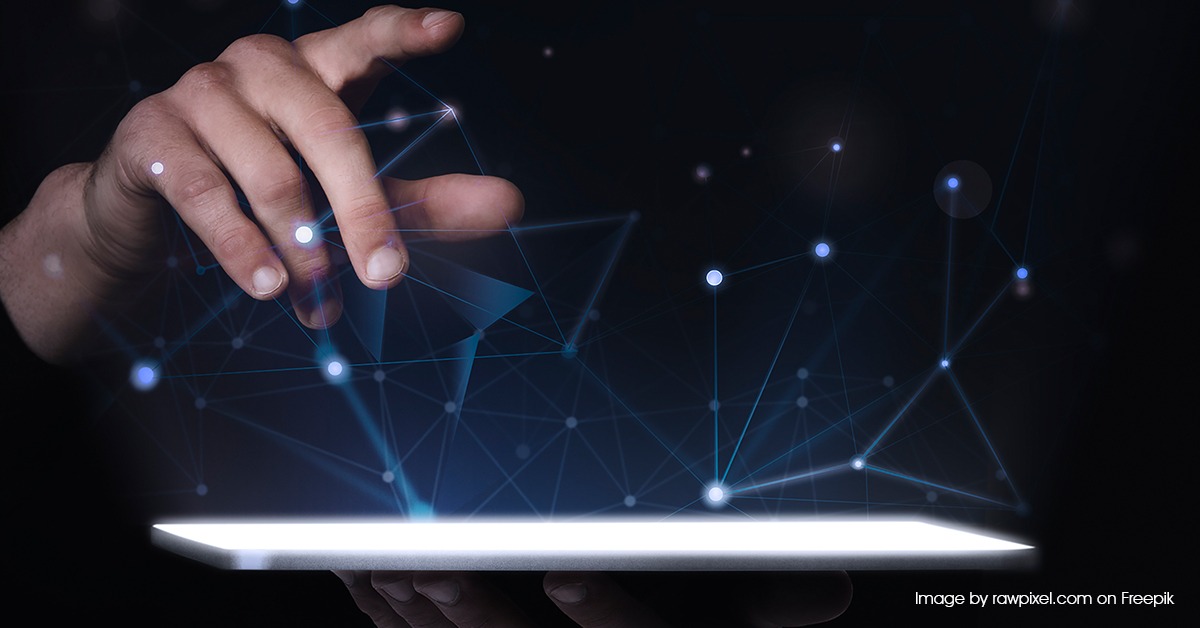
Introduction
Every organization hopes to be data-driven. Artificial Intelligence (AI) and Machine Learning (ML) allow business leaders to turn data into insights and then those insights into action. According to Genpact’s recent CIO study, in partnership with the MIT Sloan CIO Symposium, CIOs consider AI and ML the best technologies to help them achieve their business objectives. However, it is not enough only to prioritize AI and ML, the manner in which business leaders approach ML and AI needs a big change.
Moving from Experimentation to Operationalization
Business applications driven by AI and ML models enable faster and more efficient decision-making. However, the reality is that only half of all AI proof of concepts are driven to production. Since the beginning of the COVID-19 pandemic, organizations have made more effort to apply findings from AI initiatives and tie all AI investments to business value. Many enterprises also focus on modernization to gain the maximum benefit from AI capabilities. Today, AI innovation has to move from experimentation to operationalization.
Exploring Common Challenges
ML models in production need to manage large volumes of data, often in real-time. Unlike conventional technologies, AI and ML deal with probabilistic outcomes – in other words, what is the most probable or doubtful result?
Thus, the moving parts of the ML model require close monitoring and swift action when being deployed to confirm performance, accuracy, and user satisfaction.
The following factors tend to interfere with the proper development of ML models:
Data Quality
Information or data is available from multiple sources in various formats and volumes. Since ML models are built on data, completeness, quality, and semantics of data are vital in production environments.
Model Decay
In ML models, data patterns vary as the business environment changes. This evolution results in a lower ds to a lower prediction accuracy of models trained and validated on outdated data. Such degradation of predictive model performance is known as concept drift, which makes creating, testing, and deploying ML models challenging.
Data Locality
Organizations use data locality and access patterns to improve the performance of a given algorithm. But the reality is that such ML models may not work correctly in production because of the difference in the quality metrics.
These factors push ML practitioners to implement a ‘change anything and everything’ approach – but this results in more problems.
Finding a Solution
ML needs to evolve to handle these challenges. Data and analytics leaders must look for scalable and repeatable standalone software applications. Enterprise leaders must depend on Machine Learning Operations (MLOps).
MLOps help organizations accomplish automated and a highly reliable ML model deployment, consistent model monitoring, model training, quick experimentation, reproducible models, and an enhanced model deployment.
Benefits of MLOps
Implementing MLOps in an enterprise helps align business strategies, and offers various benefits such as:
- Rapid innovation: Faster and efficient collaboration among teams and an accelerated model development and deployment results in rapid innovation, allowing speed-to-market.
- Consistent results: Repeatable ML models and workflows support resilient and consistent AI solutions.
- Better compliance and data privacy: Efficient management of the ML lifecycle, data, and model lineage optimizes spending on compliance regulations and data privacy.
- High return on investment: Management systems for ML models and model metrics result in smarter spending on viable use cases.
- A data-driven culture: Data asset tracking and IT with better process quality fosters a data-driven culture.
Conclusion
While AI and ML have a place in all sectors, there is no one-size-fits-all solution to each organization’s specific problems. As the future gets more automated, organizations require a clear and holistic understanding of their data silos. This is essential to leverage the potential of AI and ML fully.