- March 2, 2022
- Posted by: Aanchal Iyer
- Category: Machine Learning
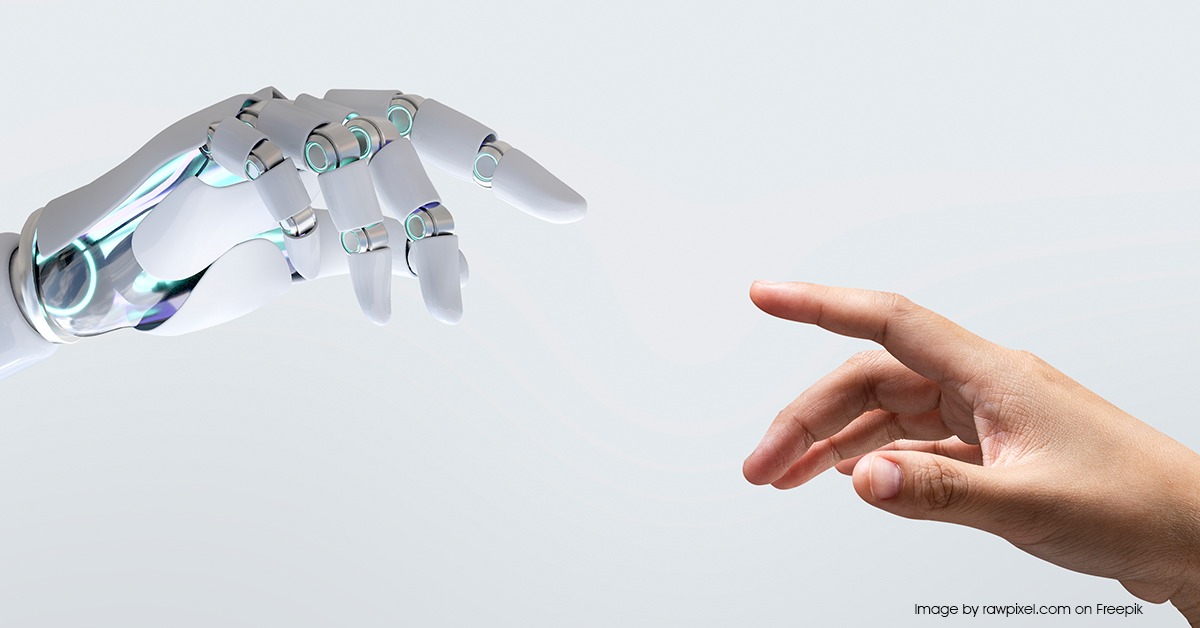
MLOPs Can Help Accelerate the Digital Transformation Process
Introduction
Machine Learning (ML) and Artificial Intelligence (AI) are no longer science fiction. MLOPs is the latest buzzword in the digitization world. It is a way to connect AI and ML to robots and labs.
Today, ML is essential for creating models capable of solving previously impossible problems. Correct implementation of the model by a professional team is the key to success. ML is now a part of the Ops family, which comprises DevOps, DevSecOps and DataOps; however, putting an ML model into production is not that simple.
What is MLOPs?
MLOPs stands for Machine Learning Operations. It offers best practices for an organization to work with AI. In other words, it is a set of tooling and best practices that allow organizations to streamline ML operations. It is a kind of DevOps for ML projects. ML operations are more complicated than software projects as they comprise more than just software code. DevOps points at a single dimension of managing code and applications, while MLOPs manage the code and the data.
Introducing MLOPs to your Organization
For MLOPs to be effective, organizations need to regulate processes across the life cycle of model development, deployment, testing, and management. Automation and ML begin by harmonizing organizational data so that all stakeholders use the same data structure. Consolidating the data and having feature stores help enable the ML models to efficiently integrate within an organization and improve velocity via reusability whether on-premises, on the cloud, or both. The direction one decides to move forward in depends on how efficiently the organization adopts ML.
MLOPs is generally an organization-wide initiative that needs strategic tools, processes, and governance to enhance value. Beyond improving the data strategy, leadership buy-in and cultural changes are essential while adopting an MLOPs culture.
The Advantages of MLOPs for an Organization
Most organizations typically ask, “how can we optimize and operationalize the ML process to accomplish more? For MLOPs, it means understanding how to make the life cycle of taking a model from lab to production faster and more efficient and gain business value to offer intelligent automation recommendations — interpreting data into meaningful business outcomes and actionable decisions.
Once an organization defines and streamlines the internal processes, data-driven intelligence can be integrated into the process automation life cycle. Integrating MLOPs in a true outcome-driven process will eradicate the manual process and enable the organization to move ML models across the applications through training, testing, and go-live. This will support the continuous development life cycle to battle model degradation. As the organization matures, its ML, the process, and automation workflows benefit from concept to realization of ML deployments.
Challenges that Organizations face While Adopting MLOPs
Organizations are using technologies, such as ML and AI, but they seem to be finding it difficult to create value. The main challenge in the ML world is around time to market and operationalization and. MLOPs tries to solve this issue by bringing in automaton via the life cycle of ML application development. As organizations embark on an MLOPs journey, the following needs to be considered and understood:
- tools and systems (infrastructure, applications, and automation tools).
- process (testing, development, monitoring, deployment, automation, and management).
- people (application development, data science, infrastructure, data engineering, and deployment).
Conclusion
For MLOPs to be successful, all facets need to work perfectly, but the biggest challenge is getting all teams together to create and agree to standardized processes and tools. MLOPs should be thought of as a journey where organizations mature iteratively where most activities are automated.