Data Science In The Banking | Top Use Cases
- April 23, 2022
- Posted by: Aanchal Iyer
- Category: Data Science
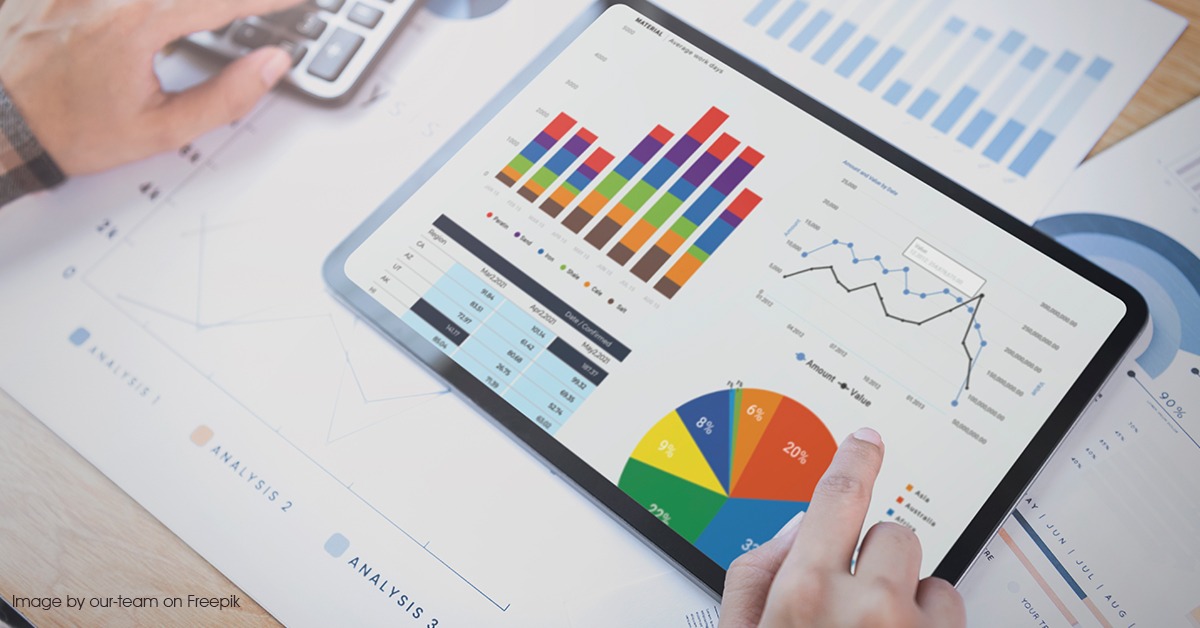
Top Data Science Use Cases in Banking
Introduction
The use of data science in the banking industry is not just a mere trend. It is indeed a necessity to keep pace with the competition. Banks have come to understand that big data technologies and data science algorithms can enable them to concentrate their resources efficiently, improve performance, and make smarter decisions.
Use Cases of Data Science in the Banking Industry
Following is a list of use cases of data science in the banking area:
-
Fraud Detection
Machine Learning (ML) is essential for effectively identifying and preventing fraud involving accounts, credit card, insurance, etc. Proactive fraud detection in banking is crucial for offering security to employees and customers. Banks can restrict account activities as soon as they detect a fraud to minimize loses. Banks can accomplish the required protection and avert significant losses by executing a series of fraud detection schemes.
-
Personalized Marketing
The key to marketing success is to create a personalized offer that is appropriate to the specific client’s preferences and needs. Data analytics allows us to develop personalized marketing that provides the right product at the right time to the right person through the right device. Data mining is broadly used for target selection to identify the potential customers for a new product.
-
Risk Modeling for Investment Banks
Risk modeling is crucial for investment banks as it enables them to regulate financial activities and plays an essential role in pricing financial instruments. Investment banking assesses the companies’ worth to facilitate mergers, build capital in corporate financing and acquisitions, perform corporate reorganizations or restructuring and for investment purposes.
-
Lifetime Value Prediction
Customer Lifetime Value (CLV) predicts the value that a business will gain from its complete relationship with a customer. This measure helps build and manage beneficial relationships with specific customers, creating more business growth and profitability.
-
Real-Time and Predictive Analytics
The ever-growing importance of analytics in banking is quite significant. Data science techniques and ML algorithms can largely improve a bank’s analytics policy as every use case in banking is carefully interconnected with analytics. As the variety and availability of information is rapidly increasing, analytics are becoming more accurate and sophisticated.
-
Customer Segmentation
Customer segmentation means creating different segments of customers based either on their specific characteristics (such as age, region, age, income for demographic segmentation), or behavior (for behavioral segmentation). There is an entire set of techniques in data scientists’ arsenal, such as decision trees, clustering, logistic regression, and so on, which as a result, helps to understand the CLV of each customer segment and comprehend low-value and high-value segments.
-
Recommendation Engines
Data science and ML tools can create simple algorithms that help segment and analyze a user’s activity to recommend them the most accurate and relevant items. Such recommendation engines demonstrate items that may interest a user, even before they have searched the items for themselves.
-
Customer Support
Customer support service is essential to keeping a productive long-term relationship with customers and is a crucial but broad concept in the banking industry. All banks are service-based businesses, so most of their activities comprise service elements. It also includes replying to customers’ complaints and questions promptly and interacting with the customers.
Conclusion
To gain a competitive edge, banks must understand the importance of data science and integrate it into their decision-making process. Banks must also develop strategies based on actionable insights from their client’s data. We can expand this list of use cases each day with a quickly developing data science field. ML models can then help to gain accurate results.