- April 7, 2022
- Posted by: Aanchal Iyer
- Category: Machine Learning
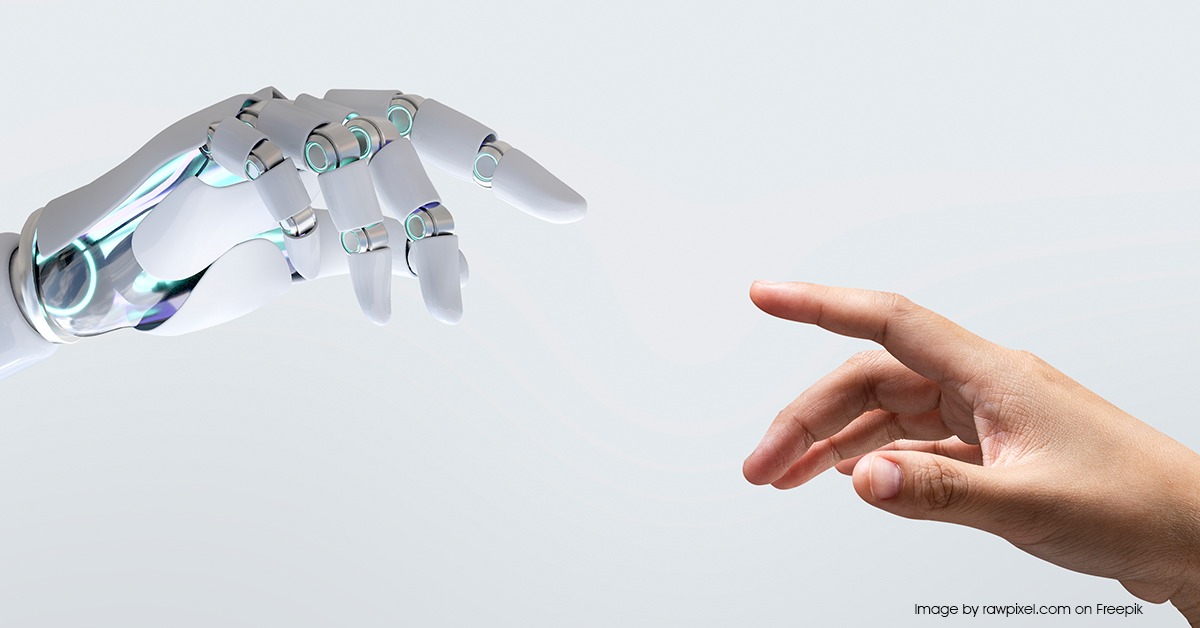
Top Machine Learning Innovations of 2022
Introduction
Machine Learning (ML) falls as a subset of Artificial Intelligence (AI) in the technology structure. Machine learning algorithms enable machines to understand data and make data-driven decisions. Some analysts predict ML will attain massive popularity by 2024, with the maximum thrust in 2022 and 2023. This blog presents the best machine learning innovations of 2022.
The fundamental reason for developing ML technology and supervised learning was to create a method that enables IT professionals and developers to quickly generate solutions and applications. New techniques in machine learning ease the job of testers working on huge volumes of variables, which probably is out of the range of human capacity.
Machine Learning Innovations of 2022
Regarding ML technology, let us look at some of the upcoming machine learning innovations in the year 2022 and beyond.
-
Internet of Things (IoT) and ML
: IoT is the first and foremost of the machine learning innovations that most IT professionals are eagerly waiting for. Development in this space will have a huge impact on 5G adoption, becoming essential for IoT. As 5G has a marvelous network speed, devices will be able to receive and transfer information faster than ever before.
-
Automated ML
: Professionals can develop effective and competent tech models that enable productivity and efficiency with automated ML. As a result, we will see most of the developments occurring in task solving. Automated ML is essentially used to create highly sustainable models that help in work efficiency. This holds good, particularly in the development space, where professionals can develop applications without programming knowledge.
-
Improved Cybersecurity
: With the various technological innovations, most the appliances and applications have become smart. However, as these smart appliances are always available on the internet, these appliances have a security requirement. With ML, tech professionals can create anti-virus models that can prevent potential cyber-attacks and minimize the risk of threats.
-
Ethics in AI
: With machine learning innovations, there comes a serious concern about describing these innovations’ ethics. The absence of these ethics will result in machines not being able to work efficiently, which will eventually lead to wrong decisions. This fact is quite evident in the self-driven cars available in the market today. The fiasco of self-driven cars is due to the failure of the inbuilt AI, which is the car’s core. There are two primary reasons:
- Developers may sometimes be partial towards choosing certain data. For instance, they utilize data where most factors are in favor.
- Most ML models fail because there is a shortage of data moderation techniques.
-
Automation of natural speech understanding process
: Automation is one of the types of machine learning techniques where huge amounts of data are shared on smart home technology, which works on smart speakers. Because of the use of smart voice assistants, such as Siri, Google, and Alexa, the process is relatively simplified and it creates a connection with intelligent appliances through non-contact control.
-
General Adversarial Networks (GAN)
: GAN is considered one of the upcoming machine learning innovations that generate samples to be checked by discriminative networks and can eradicate any type of undesirable content. Just like the government has multiple branches, GAN helps in reliability and accuracy by offering balances and checks.
Conclusion
With AI, machines had the ability to learn, memorize, and generate accurate outcomes. New techniques in machine learning will always be moving in an upward trajectory.