- June 25, 2022
- Posted by: Aanchal Iyer
- Category: Big Data Analytics
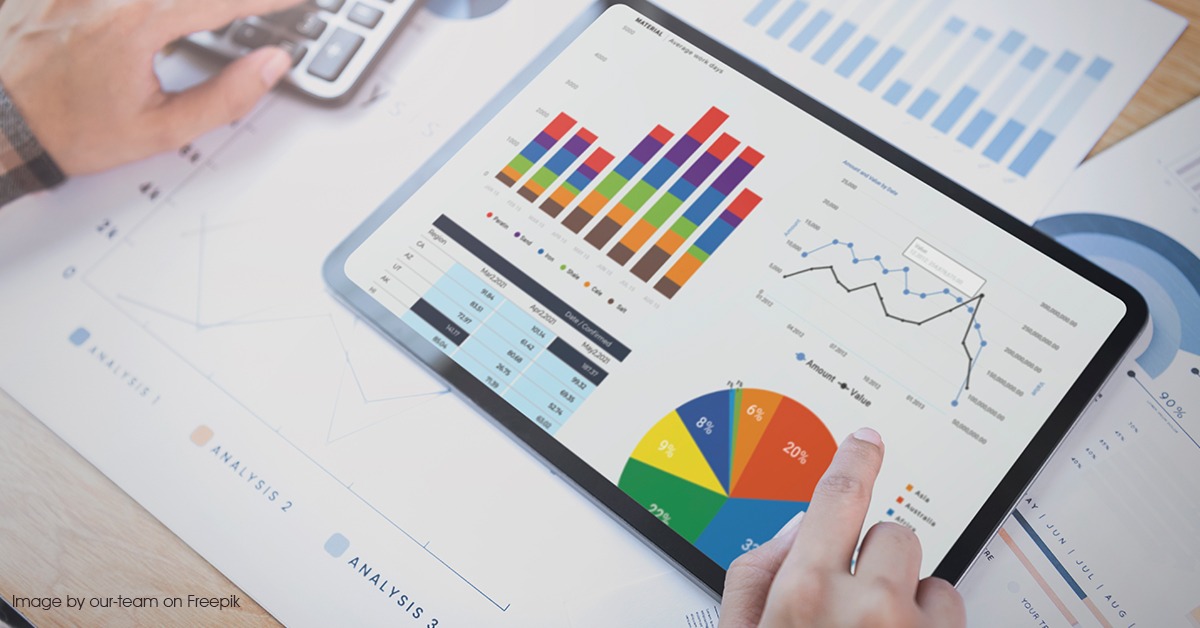
Use of Synthetic Behavioral Data in Analytics
Introduction
Behavioral analytics is a complex topic because of the nature of behavioral data. Behavioral datasets are huge, complex, and notoriously hard to disguise. These datasets are also the most valuable, comprising true Business Intelligence (BI). However, they are being increasingly hidden away for privacy reasons. Despite the enormous growth in volume in recent years, we are yet to tap into the wealth of synthetic behavioral data.
There are two key obstacles for the organizations to overcome to seize the opportunity: (i) behavioral data is complex to analyze and model since it is sequential, and (ii) behavioral data is difficult to disguise and also privacy sensitive, thus it tends to be hidden away.
Both these obstacles reinforce each other. Without safe data sharing, one cannot champion the cause of data literacy. Without data literacy, one cannot see a growing demand for synthetic behavioral data.
Behavioral Data is Essential for Data Science
Data science is gaining popularity. And, everyone seems to be moving into data science. In fact, people are changing careers, paying for online MOOCs and boot camps, and building networks on platforms such as LinkedIn. However, many such new entrants have a problem in maintaining the momentum of comprehending the new trade-craft. However, what exactly is synthetic data and why do we need it?
Synthetic data is algorithmically made approximating original data, which can be used for the same purpose as the original data. There are a few reasons behind the requirement of synthetic data. First, it can be an issue of availability. An organization or a team may not have enough data. For larger businesses, data silo systems and legacy infrastructures are often a cause of data unavailability. In today’s data protection regulatory landscape, it is also a matter of legal compliance. The data is available, but it follows a strict regulatory process. For example, the General Data Protection Regulation (GDPR) prohibits uses that were not explicitly agreed to when the organization was collating the data.
These are the reasons why organizations turn to synthetic data. Either they create datasets from partly synthetic data, where they substitute only a selection of the dataset with synthetic data. Or they use fully synthetic behavioral data with datasets that do not include any of the original data. Fully synthetic data is often available where privacy is obstructing the use of the real, original data.
Types of Synthetic Data
There are various types of synthetic data for different purposes. Synthetic data can be:
- Synthetic media like image, video, or sound: Synthetic data can also be a synthetic image, video, or sound. One artificially renders media with properties that are close to real-world data. This similarity enables using the synthetic media as a drop-in replacement for the real data.
- Synthetic text: Synthetic text is artificial text . One builds and trains a model to create text. Because of languages’ complexities, creating realistic synthetic text is always challenging.
- Synthetic tabular data: Tabular synthetic data is artificial data that imitates the real-world data available in rows and columns of tables. It could be anything ranging from a patient database to financial logs or users’ analytical behavior information.
Conclusion
Typically, all customer-facing industries can use the privacy-preserving synthetic behavioral data, as modern data procession laws regulate personal data processing.