Model Explainability is the Next Data Science Superpower! (2020)
- December 30, 2019
- Posted by: admin
- Categories: Data Science, Machine Learning
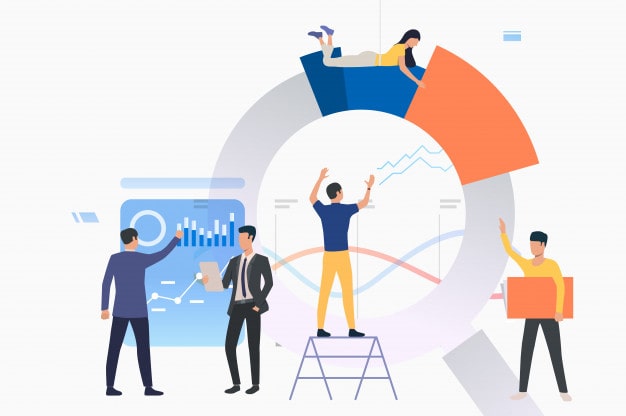
People are usually under the impression that Machine Learning techniques are only suitable for making forecasts, and in all other cases they are like black boxes – no one really understands what is going on inside. But this is indeed a misconception, for, expert data scientists can extract real-world insights from Machine Learning models easily.
High-caliber data scientists can actually explain the workings of Machine Learning models and say which features are the most important according to that model, what roles those features play in predictions and so on.
And data experts say that this kind of model ‘explainability’ is indeed highly advantageous in many ways. Out of the various applications of model insights, the following 5 have been identified to be the most important for business success.
• Debugging
Infinite volumes of data generated every day are mostly unorganized, raw data, which often play a disastrous role in real-life data science projects, and are potential sources of major errors. To reduce the frequency of such errors, debugging is considered crucial. The first step in tracking down bugs is to understand patterns in the model that will help in the identification of the areas of odds.
• Informing decision-making by humans
Certain decisions are entirely made by models and not human beings. For example, when a user visits the site of Amazon, there is no human interaction involved to help the user decide what to buy! But on the other hand, there are some decisions for which humans have to take lead, and thus model insights turn out to be much more helpful than predictions.
• Building relationships of trust
Most people find it hard to trust Machine Learning models without verifying basic facts. But if businesses attempt to show their customers insights which can help them understand the problem and relate to it, then general customers can build trust even if they have little understanding of data science.
• Informing and explaining feature engineering
Feature engineering is one of the best ways to improve Machine Learning model accuracy. This involves continuously adding new features and transformations to the raw data or to the set of features that have been previously created.
But at times when hundreds of features are already present or the user lacks sufficient knowledge about the topic, things start looking confusing. It is during these times that enough knowledge about the background topic becomes mandatory or in complex cases, help from a professional is suggested.
• Directing future data collection
As a user, one doesn’t have any control over the datasets that are downloaded from online sources. But various organizations are now creating opportunities to diversify the types of data they collect by using different data science techniques. In most cases, collecting new types of data turns out to be inconvenient and expensive, so they only proceed if they have an idea that the prediction of the process will be worthwhile. Mode based insights will not only help you to get a clear understanding of the set of features you already have, but it will also help you determine that if adding in new features will improve the value of your business.