- January 25, 2022
- Posted by: Aanchal Iyer
- Category: Deep Learning
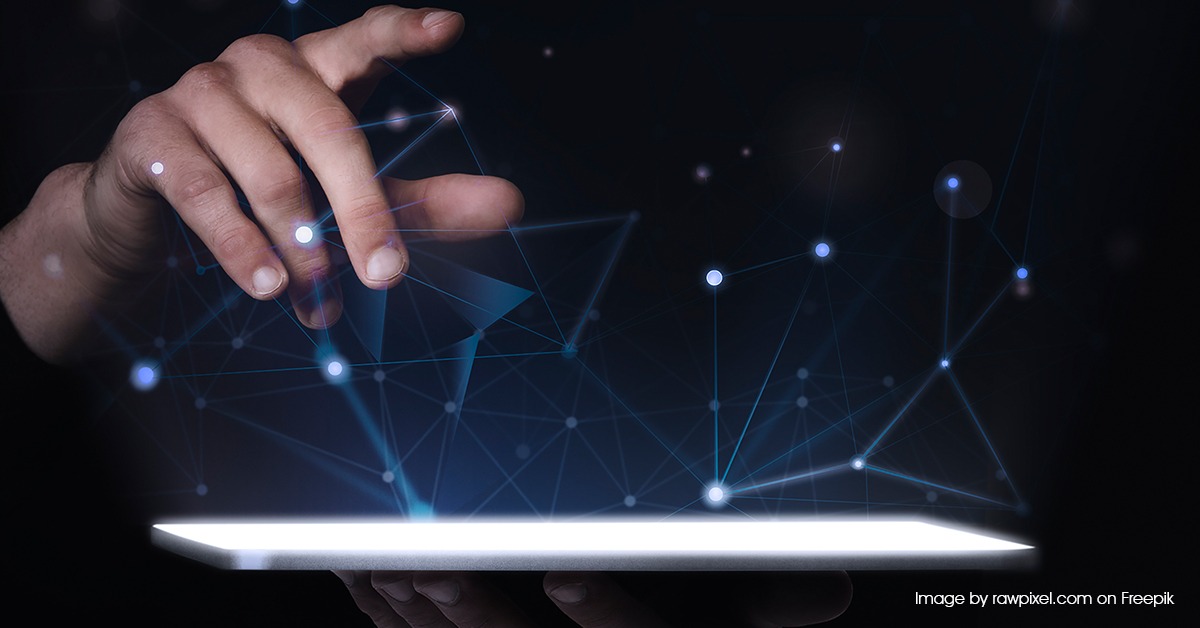
Deep Learning in the Healthcare Industry
Introduction
Let us first understand what deep learning is. Deep learning is a potent tool that identifies patterns of specific conditions that develop or arise in our body, a lot faster than a clinician. Deep learning imitates the working of the human brain through a pattern of data inputs, biases, and weights. Making accurate predictions and clustering data are the primary mechanism of deep learning.
Reasons for Making a Paradigm Shift from a Traditional Approach to a Machine-Driven Approach
The quality of patient results in a typical hospital setup is not good. Following are the reasons from a traditional approach to a machine-driven approach:
- We do not have sufficient clinician workforce to help with the increasing population with varying conditions.
- It is challenging to scale up clinicians, however, it is easy to scale up machines.
- There is a consumerism approach in private hospital setups that affect the quality of patient results.
- The government-controlled hospitals have administrative bottlenecks that result in poor health outcomes.
A machine-driven approach is independent of all the above limitations, thus, having the potential to display better results.
Uses of Deep Learning in Healthcare
Deep learning is steadily finding its way into innovative tools with high-value applications in the real-world clinical environment. Some of the most promising use cases comprise innovative patient-facing applications and strategies for enhancing the health IT user experience.
-
Imaging Analytics and Diagnostics
Convolutional Neural Networks (CNNs) is a deep learning tool that analyzes images, such as x-rays or MRI results. CNNs designed with an assumption that they will aid in processing images, enabling the networks to function more effectively and handle larger images. As a result, some CNNs even surpassing the precision of human pathologists while identifying essential features in diagnostic imaging studies.
-
Natural Language Processing
Neural networks and deep learning already highlight most of the natural language processing tools popular in the healthcare industry for managing documentation and changing speech-to-text.
As neural networks are created for categorisation, they can recognize specific linguistic or grammatical elements by arranging related words together and plotting them in relation to one another. This helps the network understand complex semantic meaning.
-
Precision Medicine and Drug Discovery
Drug discovery and precision medicine are also on the map for deep learning developers. Both tasks need to process vast volumes of clinical, genomic and population-level data. This data needs to be processed to identify undetermined associations between pharmaceuticals, genes, and physical environments. Deep learning is the perfect strategy for pharmaceutical stakeholders and researchers looking to emphasize new patterns in these unfamiliar data sets.
-
Predictive Analytics and Clinical Decision Support
The industry hopes for deep learning in predictive analytics and clinical decision support for various conditions. Deep learning may very soon become a diagnostic acquaintance in the inpatient setting, where it can warn providers about changes in high-risk conditions, such as respiratory failure and sepsis.
Challenges of Deep Learning in the Healthcare world
- Insufficient data results in low accuracy
- Issue of interpretability with deep learning models makes it look like a black box.
- Limitation of data for rare diseases
Conclusion
The healthcare segment is changing from paper-based records to Electronic Health Records (EHR). This will open the floodgate of opportunities for the application of deep learning in this field. Patient-clinician interaction will also improve, resulting in better health outcomes in a hospital setup. Deep Learning is the key to the success of clinicians in the field of medical science in future.
Our service is our USP. Try our mix and match range to believe it.