Green Machine Learning
- November 18, 2022
- Posted by: Aanchal Iyer
- Category: Machine Learning
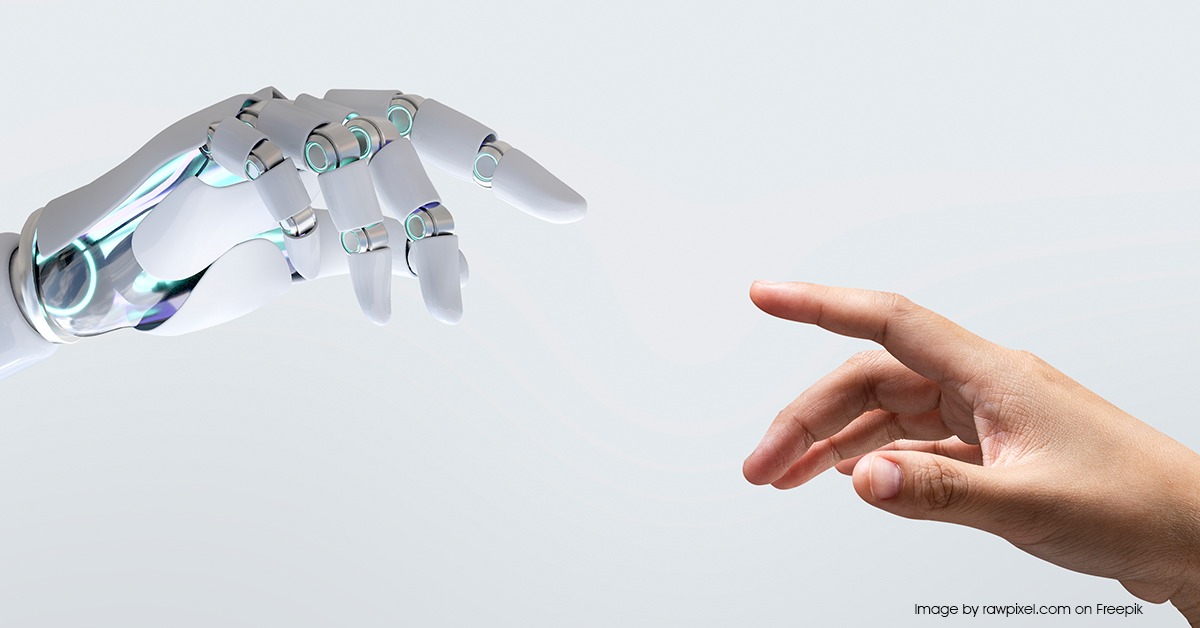
Introduction
The impact of Artificial Intelligence (AI) and Machine Learning (ML) on the environment has been a hot topic and will be the most defining issue of AI and M for this decade. This conversation started with some recent studies from the Allen Institute for AI. The argument was for the prioritization of “Green Machine Learning (ML) and AI” efforts that concentrate on the energy efficiency of AI systems.
Green Machine Learning (ML) and Green AI are topics that have gained a lot of attention as it appeals to researchers to concentrate on carbon emission and energy usage during inference and model training.
More on Green AI and Green ML
As industries adopt AI and ask for more powerful and sophisticated AI models, this demand will only grow in the future. A huge amount of computational cost and energy is required to train AI models, raising worries about its carbon footprint. According to some recent studies, the amount of computing power for advanced AI training has grown exponentially since 2012.
Thus, we have to develop sustainable AI models to gratify the existing demand.
Green ML and AI refers to machine learning and artificial intelligence that is environment friendly. Its objective is to accomplish sustainability through environmentally sustainable AI models with much less carbon emissions and computational costs. Green AI can have positive impacts in various sectors. For example, Al can predict requirement and supply in the energy sector. This can enable an intelligent grid system that reduces energy wastage. For example, Google uses AI to enhance energy efficiency, leveraging DeepMind’s ML capabilities to decrease the amount of energy needed to cool its data centers by around 40%.
Moving your Organization Towards Green AI
When technology can offer more sustainable options, you should embrace it. Here are a few ways to adopt Green machine learning and Green AI
- Enhance reproducibility: Sharing and reproducibility of transitional information is critical to increase the efficiency of AI development. Often, AI research are published without any source code. Usually, researchers fail to reproduce same results even on obtaining source code. Such factors are main drivers of Red AI as they cause duplication of efforts and stop efficient sharing.
- Enhance hardware performance: There is a plethora of progressive hardware that offers better performance on deep learning tasks enhancing reliability. Upgrading to performance-based hardware reduces the usage and cost of energy.
- Democratize and understand deep learning: Deep learning is effective. Detecting the fundamental science of AI and ML and formally defining its shortcomings and strengths aids in creating reliable and practical models. Prevailing models are now accurate enough to be used in multiple applications. ML tools can support most businesses.
- As AI ad ML becomes widespread, energy efficiency is even more crucial. There are much bigger levers for decreasing carbon emissions, such as moving away from coal power.
- Partner more: The largest organizations in the world do not have the talent to build AI competently. However, their leaders understand that ML and AI will be main components of future services and products. Organizations should look for partnerships with incubators, startups, and universities to kickstart their AI and ML strategies.
Conclusion
We have seen extraordinary strides in AI and ML in the recent years. However, we are only in the early stages in terms of sustainability. It is crucial to concentrate on the best methods, systems, and methodologies for building efficient AI models. With the right technology and approach consultation, it is possible to create AI tools that are powerful and sustainable. As AI research grows, we must demand that the best tools, platforms, and methodologies for creating models are reproducible and easily accessible.