Introduction to Data Lakes
- May 27, 2022
- Posted by: Aanchal Iyer
- Category: Data Science
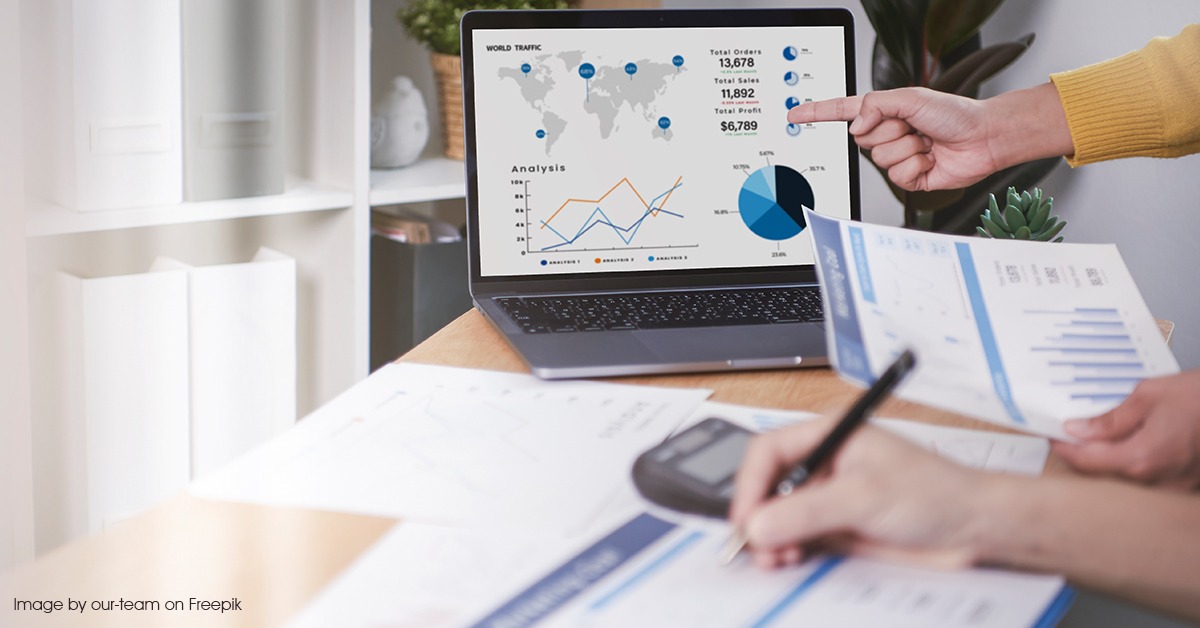
Introduction
A data lake is nothing but a central repository that enables the storage of structured and unstructured data at any scale. Structured data is data or information that is highly organized, to-the-point, and factual. Unstructured data does not have any structure to it and comes in all its diversity of forms.
In a data lake, one can store their data as it is without having to worry about structuring the data first and executing various types of data analytics.
Data Lakes Vs. Data Ware Houses
An organization generally requires both a data lake and a data warehouse, since both these approaches serve different requirements and use cases.
A data warehouse is an improved version of a database to analyze the relational data that comes from the line of business applications and transactional systems. The schema and data structure are clear beforehand. This helps in the execution of fast SQL queries – where the results are used for analysis and operational reporting.
A data lake stores non-relational data from IoT devices, social media, and mobile apps and relational data from the line of business applications. The schema and data structure are not clear at the time of the data capture. This means that a data lake allows for data storage without the need of any careful design. One does not even have to worry about the answers to the questions related to the data which may arise in the future.
The Vital Elements of a Data Lake and Data Analytics Solution
As organizations are setting up data lakes and an analytics platform, they need to think about the multiple advantages of such a platform. The advantages are:
-
Democratize Data: A data lake can make data available to the whole organization. It is what we call data democratization.
-
Get Better Quality Data With the tremendous processing power of a data lake, one can use tools to ensure the data is of good quality.
- Storage of data in the native form: There is no need for data modeling at the time of data capture.
- Scalability: It provides scalability and is fairly reasonable as compared to a conventional data warehouse when we consider the factor.
The Importance of a Data Lake
The skill to utilize more data in less time from more sources, and empower users to analyze and collaborate data in different ways results in faster and better decision making. Examples where data lakes have added value are:
- Better customer interactions
- Better R and D innovation choices
- Enhanced operational efficiencies.
The Challenges of Data Lakes
The key challenge with respect to a data lake architecture is the storage of raw data. Well-defined mechanisms to secure and catalog the data have to be present in the data lake.
Without such elements, the data cannot be fully trusted, resulting in a “data swamp.” Data lakes need to have semantic consistency, governance, and access controls.
Conclusion
The cloud is ideal for the deployment of a data lake. The cloud offers scalability, reliability performance, a diverse set of analytic engines, and availability. Top reasons why the cloud is the best for data lakes are:
- Security
- Faster time for deployment
- Better availability
- Better functionality/feature updates
- Geographic coverage