Use of Federated Machine Learning Effective in Financial Crime Data
- September 26, 2022
- Posted by: Aanchal Iyer
- Category: Machine Learning
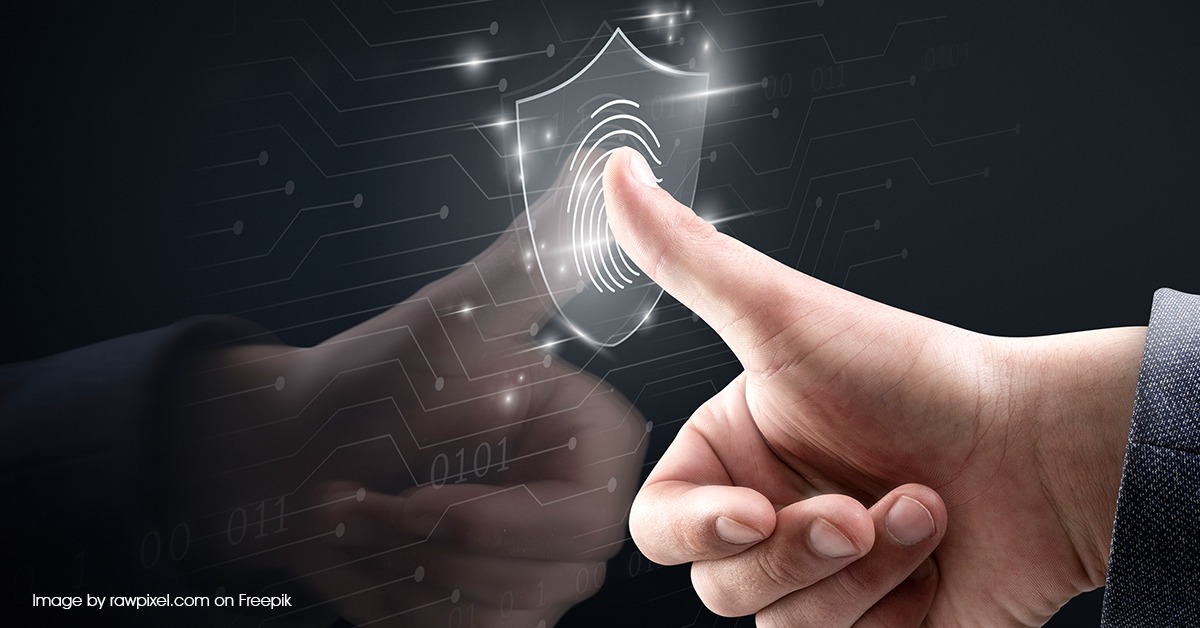
Use of Federated Machine Learning Effective in Financial Crime Data
Introduction
Federated Machine Learning (ML) uses decentralized development processes and model training. In federated ML, a classification or learning algorithm trains on data that node entities store. These node entities are the participating financial institutions in the federal learning process. In such a process, each node lets the training data available on its servers to access certain algorithms. These nodes then upload the updates of the algorithm to a coordinating server. In contrast, non-federated forms of ML, generally train on data held on a central server, which can be impractical, costly, or risky to collate due to confidentiality requirements and competitive considerations.
More on Federated Machine Learning
The financial industry is well aware that AI can change and help in a lot of ways. However, the industry cannot just jump into AI/ML without resolving the challenges and concerns regarding the same.
For many reasons, which also include sovereignty, privacy, and the cost of moving data — the financial industry does not share data between institutions. Data remains isolated within banks and within the departments within banks, restricting the amount of data that is really available for training ML. Now, imagine a scenario where data and expertise can be easily shared between communities of professionals while enhancing privacy. This is exactly the world of Federated Learning. Instead of pooling data from different financial institutions for training ML models, moving computations to the data (stored at the financial institutions) securely is a much better approach. This new approach is the foundation of federated learning.
How can Federated Machine Learning Help the Financial Industry
Federated learning, the next big thing in ML, resolves these data challenges. It aims to bring AI/ML systems to extraordinary standards of efficiency and effectiveness. The present system, forces the financial industry on spending billions of dollars on compliance, however, counters less than 1 percent of the problem, thus, making it quite incompetent.
AI and ML are providing the latest technologies to transform the recognition of terrorist financing, money laundering, and other financial crimes, making it both efficient and effective. ML technologies learn by example (inductive learning). Given large enough examples of the various types of financial behaviors, ML algorithms can recognize and distinguish the learned behavior from all others. For example, when the model is trained on a cat, a machine can differentiate images of cats from all other non-cat images. When an algorithm trains on a certain type of financial crime behavior or bank customer, a machine can differentiate between the learned customer behaviors from the behaviors of the rest.
Federated Machine Learning is more Accurate
Federated ML is precise, for a variety of reasons:
- It removes information-sharing restrictions. By federating ML, collaboration happens without the sharing of any data.
- It discovers more illicit activities. Through collaboration, model effectiveness improves beyond the upper bound of any single bank’s ability to create its own model, ML or otherwise.
- It works faster and at lower cost. Using federated ML, banks can send fewer cases for human review and in turn the amount spent on fines, which is probably the best way to control costs.
Wrapping Up
When we refer to banks and their data, we typically refer to data islands – since the instant you move that data beyond the enterprise, compliance and privacy risks emerge. Federated machine learning is here and ready to change this game. The privacy and security that is inherent to federated learning enable an increase in effectiveness and efficiency. This technology has the potential to transform not just the conventional banking industry but also the fintech industry and online payment platforms.