Automatic Speech Recognition (ASR) – How it Works?
- February 8, 2022
- Posted by: Aanchal Iyer
- Category: Machine Learning
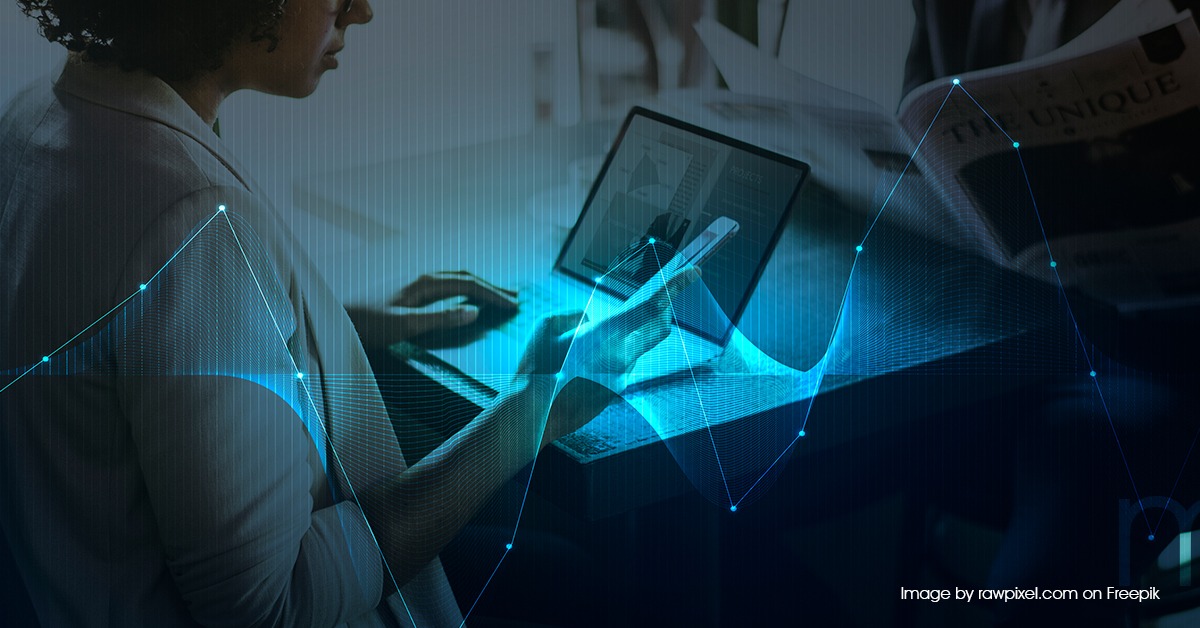
Automatic Speech Recognition (ASR) – How it Works?
Introduction
In the world that we live in today, Machine learning (ML) and Artificial Intelligence (AI) are so prevalent and helpful that most individuals use these technologies in their daily lives without giving it much thought. One main area where these smart technologies have advanced significantly, almost to a point where they are equal to human abilities, is the field of Automatic Speech Recognition (ASR) technology.
ASR Technology
Over the last decade, Voice Assistants have turned universal with the popularity of Amazon Echo, Google Home, Cortana, Siri, and many others. These are some of the best-known examples of ASR technology. This class of applications begins with a clip of verbal audio in a particular language and cites the spoken words as text. It is for this reason they are known as Speech-to-Text algorithms.
Of course, applications, such as Siri and the others go further. These applications extract the text but and interpret the semantic meaning of the spoken words, in a way that they can respond with answers, or take actions corresponding to the user’s commands.
How ASR Technology Works
Developments in AI in combination with the global pandemic have encouraged businesses to improve virtual interactions with their customers. Thus, businesses are increasingly turning to chatbots, virtual assistants, and other speech technology to efficiently control these interactions. The basic ASR programs today still use directed dialogue, while the advanced versions use the AI subdomain of Natural Language Processing (NLP).
-
Directed Dialogue ASR
: These ASR programs understand only short, simple verbal replies and in turn have a limited set of responses. These are useful for short, forthright customer interactions but not for complex interactions.
-
Natural Language Processing-based ASR
: NLP is a subdomain of Artificial Intelligence. It is the method of teaching computers to comprehend natural language or human speech. Following are a few points to understand the working of the NLP:
- One states a command or asks a question to the ASR program.
- The program then converts the speech into a spectrogram – a machine-readable representation of the audio file of the words.
- An acoustic model cleans up the audio file by removing any background.
- The algorithm breaks down the cleaned-up file into phonemes – the basic building blocks of sounds.
- The algorithm analyzes the phonemes in a sequence.
- An NLP model then applies context to the sentences.
- Once the ASR program understands what you are trying to say, it can then develop a suitable reply and use text-to-speech conversion to reply to you.
Automatic Speech Recognition Applications
Following are a few ASR applications that stand out:
-
Voice-enabled Virtual Assistants
: There are various popular virtual assistants, such as Apple’s Siri, Google Assistant, Amazon Alexa, and Microsoft’s Cortana.
-
Transcription and Dictation
: Many industries depend on speech transcription services. These services are useful for transcribing customer phone calls in sales, company meetings, investigative government interviews, and capturing medical notes for a patient.
-
Education
: ASR provides a useful tool for education purposes.
-
In-car Infotainment
: In the automotive industry, ASR is being used to provide an improved in-car experience.
-
Security
: ASR can provide improved security by requiring voice recognition to access certain areas.
-
Accessibility
: ASR also serves as a promising tool for advancing accessibility.
Conclusion
Though ASR does pose a host of challenges, most of these challenges can be overcome by using customized data collection and annotation project. The right data partner can connect you with the data you need for your particular use case and help you quickly launch with their data platform, and be inclusive with your ASR application.