- January 30, 2023
- Posted by: Aanchal Iyer
- Category: Data Science
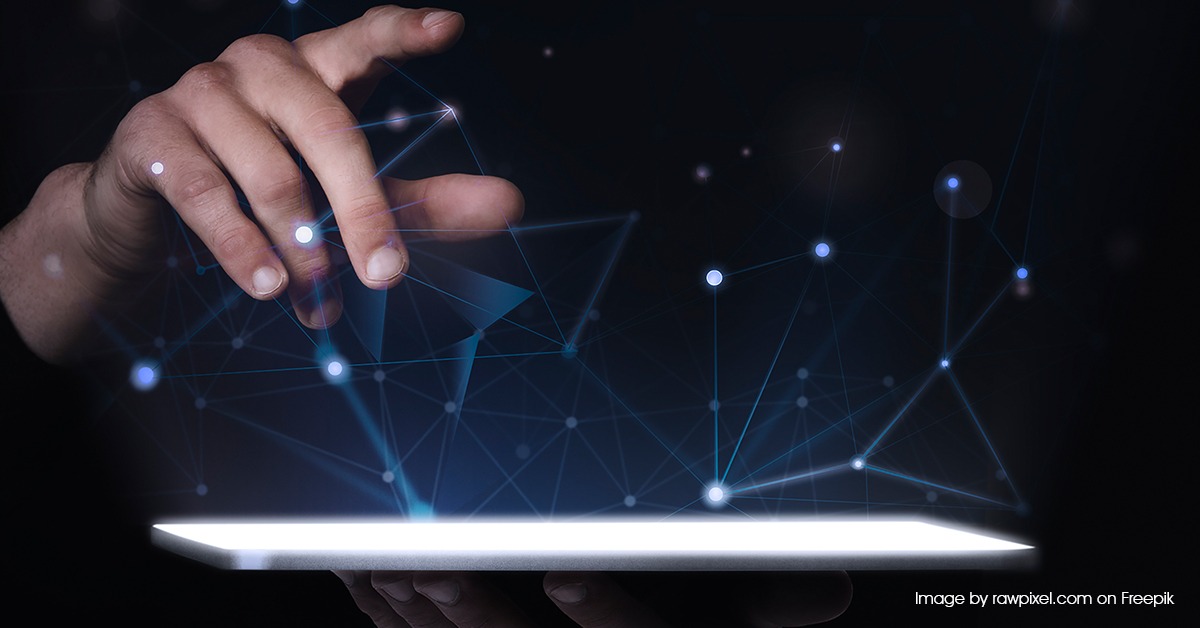
Introduction
Management of and connecting data science talent and their respective skills with data analytics projects is a prevalent business challenge. However, supporting and scaling data science abilities necessitates an entire range of skills and an understanding of how specific roles can best offer support to the business.
An all-inclusive data science team can help in establishing data science projects that are purposeful and productive. Data science skills may vary depending on a specific project or business question. When there is an understanding of how specific roles and responsibilities align with business objectives, it helps team members provide a more successful result.
Benefits of a Great Data Science Team
Below are just a few reasons why the right data science team is beneficial:
- Ensures addressing of the right business problems.
- Suitable data analysis can enhance cost efficiencies and revenue, and target opportunities for excellent business growth.
- Scaling of algorithms is possible in production.
- Data insights can support leadership and empower business users.
- Agile integration of various data sources.
- Improves decision-making and infuses data and analytics into the organization’s DNA.
- Helps in understanding the market trends and the corresponding impact on operations.
- Helps in better linking of data insights to business actions.
Steps to Creating a Data Science Organization that Delivers ROI
Ensure that you implement the following steps to create a data science organization that delivers ROI:
Focus on Business Areas that Are Ready
To set realistic expectations and create a sustainable ROI, ensure to start small. You can take the first step in your journey by looking at areas where there is opportunity and excitement waiting. Focus on the few parts of the business that understand the value of data science. Look for areas where clear, data-oriented, and measurable questions are present and can be solved.
Build context: Align Technical Teams to the Business they Support
Context is essential to setting technical talent up for success. The farther away a data science team is from the business, the more likely they will miss valuable insights. One must ensure to align data science directly to the business area that the organization supports by creating cross-functional teams. This structure can enable critical connections between the teams closest to the data and the business partners they support.
Keep it simple: Opt for quality over complexity
It can be tempting force your team to begin cutting-edge machine learning approaches – but complex science does not always mean better science. Take time to frame business problems clearly rather instead of diving into complex modeling and AI. High-quality and simple data assets allow a team to answer critical questions with confidence.
Drive scale: Develop Advocacy for the Power of Data Science
Once a data science organization begins to deliver value by starting small, one can scale that value across the business. For example, a data science team has identified the correct price points for a certain brand to enhance consumer engagement. Analysis is done and prices have been modified and ROI measured. Now, one can drive scale by applying that approach to another brand in the portfolio.
Conclusion
Data science models, just as software in general, require much future effort due to the need for maintenance and upgrades. They have an extra layer of complexity and effort because of their dependence on data and the resulting requirement for retraining. Logging everything and retraining models periodically are effective ways to address these issues.